Eye Tracker Allows Users to Control Robotic Arm
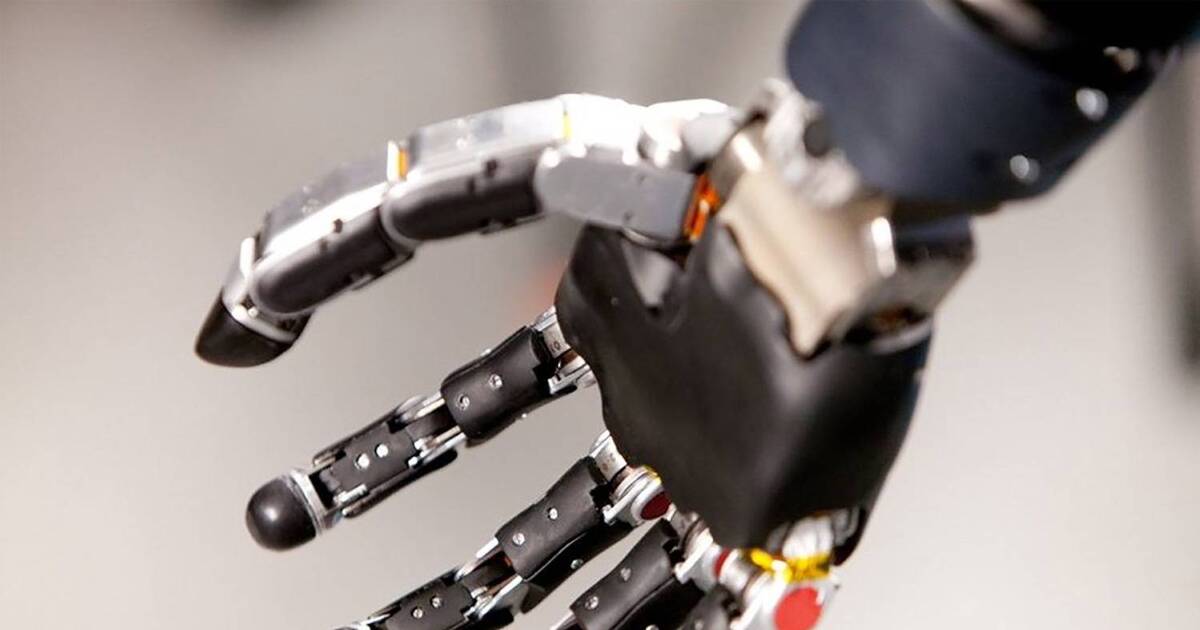
Researchers from the Georgia Institute of Technology (Georgia Tech) and Washington State University have developed an intelligent two-camera eye-tracking system (TCES) that represents a significant step forward in how humans interact with robots, the journal Advanced Intelligent Systems reported.
This work, led by Woon-Hong Yeo, Woodruff Faculty Fellow and associate professor at Georgia Tech, provides a hands-free, high-precision, remote control of complex robotic systems by detecting a user’s eye movements.
The existing eye-tracking systems show limited accuracy and data loss caused by user’s movements and skin-mounted sensor quality. These limitations are obstacles to high-precision detection of eye motions for persistent human-machine interactions.
“To solve the current problems, we developed the TCES that uses two cameras with an embedded machine learning algorithm for more accurate eye detection,” said Yeo.
As a result, the TCES shows the highest accuracy compared to any other existing eye-tracking methods. In this study, the researchers demonstrate that this system can accurately and remotely control a robotic arm with over 64 actions per command.
According to Yeo, the TCES has broad applicability for further development of eye-tracking technology for practical applications, making it a vital contribution to human-machine interfaces.
For example, this system can find an application for medical beds, allowing patients to call a doctor or nurse or control medical equipment without using their hands. Additionally, the TCES interface could assist surgeons by providing extra maneuvering tools when both hands are occupied. The system could also be utilized in construction sites or warehouses to control heavy equipment. Using the TCES, heavy equipment can lift heavy boxes repetitively, making the process more efficient and safer for workers.
Overall, this human-machine interface has the potential to assist in various industries and improve quality of life for many people, according to Yeo.
TCES utilizes an embedded deep-learning model to monitor a user’s eye movement and gaze. The system can classify eye directions, such as up, blinking, left, and right, by training the algorithm using hundreds of eye images.
The trained model uses an eye tracker to control the robotic arm via an all-in-one user interface. In the control software, a user’s eye works like a computer mouse, controlling movement by looking at multiple grids and making eye commands to show intention. This feature allows the user to issue various commands to the robotic arm within its operating range without risk of control issues.
The team says their work has the potential to revolutionize the way we think about controlling robotic systems, and that they hope to one day integrate it with wearable prosthetics and rehabilitation devices to offer new opportunities for people with physical disabilities.
“Collectively, the TCES represents a significant advancement in the field of human-machine interface technology and is poised to transform the way we interact with machines in the future,” concluded Yeo.
4155/v