New AI Model to Prevent Damaging Data Breaches
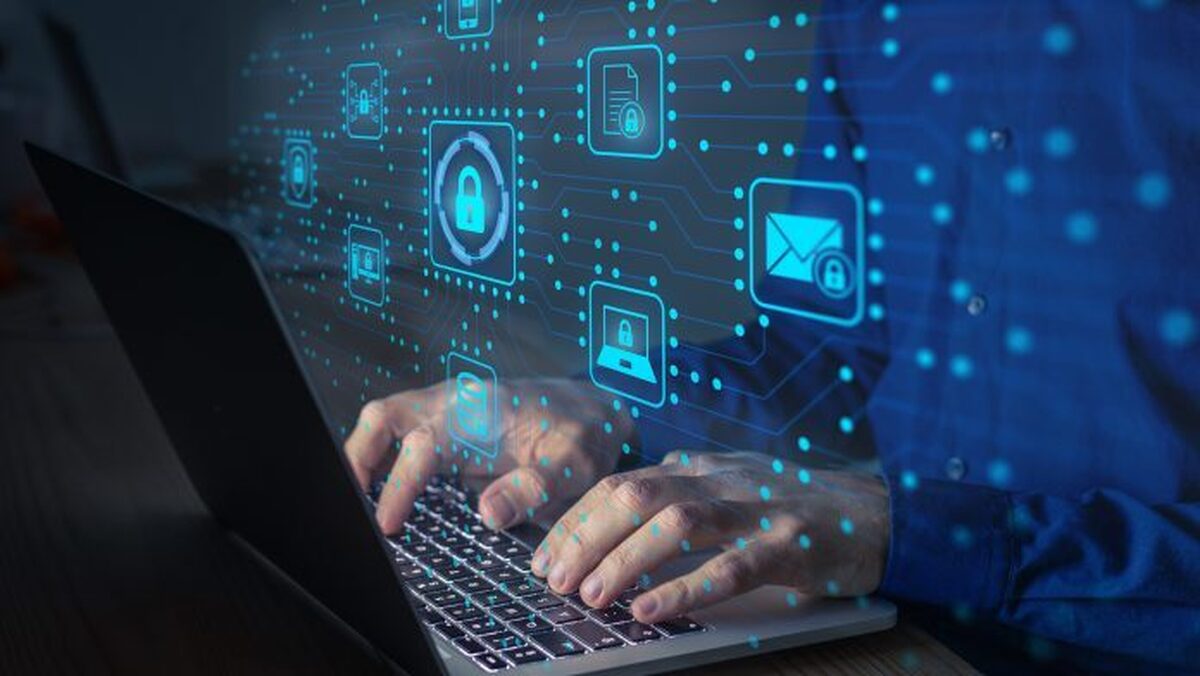
The team analysed data breaches on QBS systems – controlled interfaces through which analysts can query data to extract useful aggregate information about the world, the journal Computer and Communications Security reported.
Through this, they were able to develop a new AI-enabled method called QuerySnout. This is the first time AI has been used to automatically discover vulnerabilities in this type of system.
Dr. Yves-Alexandre de Montjoye, a senior author of the study, said, “Attacks have, so far, been manually developed using highly skilled expertise. This means it was taking a long time for data breaches to be discovered, which leaves systems at risk.
“QuerySnout is already outperforming humans at discovering vulnerabilities in real-world systems.”
The ability to collect and store data has greatly increased over the last decade. Despite this data being helpful in driving scientific advancements, most of it is personal, hence why its use raises serious privacy concerns. Laws such as the EU’s General Data Protection Regulation aim to prevent serious data breaches regarding personal information.
This means that enabling data to be used for good, while protecting our fundamental right to privacy, is a timely and crucial process for data scientists and privacy experts.
QBS systems have the potential to enable privacy-preserving anonymous data analysis at scale. Curators keep control over the data, therefore meaning they can check and examine queries sent by analysts to prevent data breaches.
However, this system is flawed, as illegal attackers can bypass these systems by designing queries to infer personal information. They gain specific people’s information by exploiting vulnerabilities or implementation bugs in the system, resulting in serious data breaches.
The risks of unknown strong ‘zero-day’ attacks, where hackers capitalise on system flaws, have stalled and delayed the development of QBS systems. To test the strength of these systems, data breach attacks can be simulated in order to detect information leakages and identify possible flaws.
However, manually designing and implementing these attacks against complex QBS is a difficult and lengthy process. Therefore, according to the researchers, limiting the potential for security attacks is essential to enable QBS to be used safely.
QuerySnout works by learning which questions to ask the system in order to gain answers. It then learns to combine the answers automatically to detect potential privacy vulnerabilities.
By using Machine Learning, the model can create a data breach consisting of a collection of queries. These queries combine answers to reveal pieces of private information using a fully-automated technique called ‘evolutionary search’, enabling the model to discover the right set of questions to ask.
Because the process takes place in a ‘black box setting’, the AI only needs to access the system rather than know how it works in order to detect potential data breaches.
Ana-Maria Cretu, co-first author of the study, said, “We demonstrate that QuerySnout finds more powerful attacks than those currently known on real-world systems. This means our AI model is better than humans at finding these attacks.”
Presently, the QuerySnout system only tests a small number of potential data breaches. Therefore, the team is seeking to advance the system further to detect even more complicated vulnerabilities.
According to Dr. de Montjoye, “The main challenge moving forward will be to scale the search to a much larger number of functionalities to make sure it discovers even the most advanced attacks.”
Despite this, the model can enable analysts to test the robustness of QBS against different types of attackers. The development of QuerySnout represents a key step forward in securing individual privacy in relation to query-based systems.
4155/v