Artificial Intelligence Could Be Promising Alternative to Animal Models
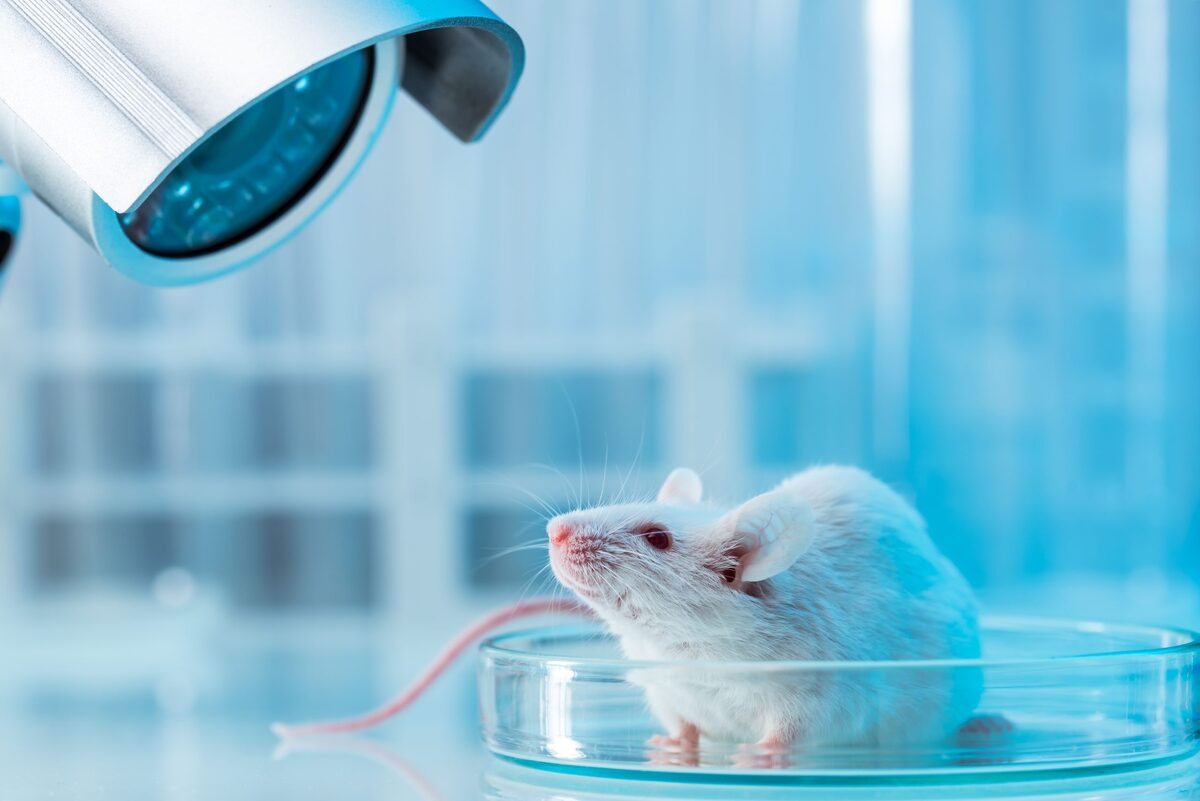
The use of animals in science experimentation is a double-edged sword: this research has saved countless human lives and has advanced our scientific understanding, but at the same time, has come at a high cost paid by the animals involved, the Advanced Science News reported.
Animal testing has been carried out for centuries, with the first references made as early the 3rd and 4th centuries BC in the writings of Greek physician-philosophers such as Aristotle (384-322 BC), who used animals to reveal important internal features in mammals. Without animal tests, it would be hard to imagine the advent of life changing medical innovations, such as vaccines, antibiotics, drugs like insulin to treat diabetes, and a host of others.
With the advent of genetic engineering, scientists have been able to better model human diseases and disorders such as diabetes, Alzheimer’s disease, various cancers, and Parkinson’s disease, to name just a few.
While immensely important from the standpoint of modern medicine and research, the fact is that in recent years in the European Union alone, 9 million animals are used every year in the lab.
This data is disturbing and is the subject of continuous controversy due to the ethical implications related to the suffering of these animals, giving rise to many initiatives against animal testing and in favor of alternative methods.
Aside from the complex ethical questions being raised, there is also the concern regarding the accuracy of animal models in mimicking what a specific drug would do in a human body, especially when it comes to mimicking toxicology.
Data actually shows that when extrapolated to humans, many animal models have limited reliability when it comes to predicting how a drug will behave. This leads to time and money wasted and unnecessary suffering during drug discovery as a drug will perform well in animal models and then fail in clinical trials or vice versa.
Even within the context of toxicity tests for new drugs or chemical compounds, there is growing pressure to avoid animal experimentation. This is something positive if we take into account that we must seek a balance between our own development and our responsibility to act as stewards of our planet (see for example the European Union REACH/3R principles, as well as Tox21 and ToxCast by the US government).
One solution is to use computer models instead — a field which has grown exponentially in recent years and integrates the perspectives of statisticians, toxicologists, biologists, chemists, engineers, and mathematicians to analyse existing data and generate reliable predictions. This was explored in a recent article published in the journal WIREs Computational and Molecular Science.
With the enormous progress made within the realm of artificial intelligence (AI) and its application in many fields such as medicine and chemistry, this alternative to animal models is revealing its full potential. This is especially evident with new deep learning techniques that are capable of self-learning using millions of known chemical compounds and data collected in a growing number of freely accessible databases which allows them to predict how a new substance will behave in humans or in the environment, thus boosting already impressive predictive capabilities.
Early in the pandemic caused by the SARS-CoV-2 virus, a group at BenevolentAI identified a potential therapeutic for COVID-19 using their machine-learning approach, which scoured the medical literature and known disease mechanism. As a result, the kinase inhibitor baricitinib, used commonly to treat arthritis. It was selected because of its effects on endocytosis, which hypothetically could be used to also disrupt entry of the virus into healthy cells. It was given emergency authorization by the FDA and used in clinical trials, showing a mild degree of improvement in COVID-19 patients. The question among experts is, does the drug work via the mechanism predicted? How accurate was this search and find mission?
Regardless, this was an exciting example of data base searches to identify potential drug candidates to solve pressing real-world problems without having to run animal studies first. Granted, this example did not completely circumvent the need for animals as baricitinib was tested in such models before being used to treat arthritis, but the hope is that one day we will get there.
These preliminary applications are just the beginning, and with quantum computing now making waves with recent breakthroughs and enhanced computational capabilities, deep learning applications will become better and better, allowing us to move away from a difficult practice.
4155/v