Iranian Researchers Design System for Real-Time License Plate Detection Using Convolutional Neural Network
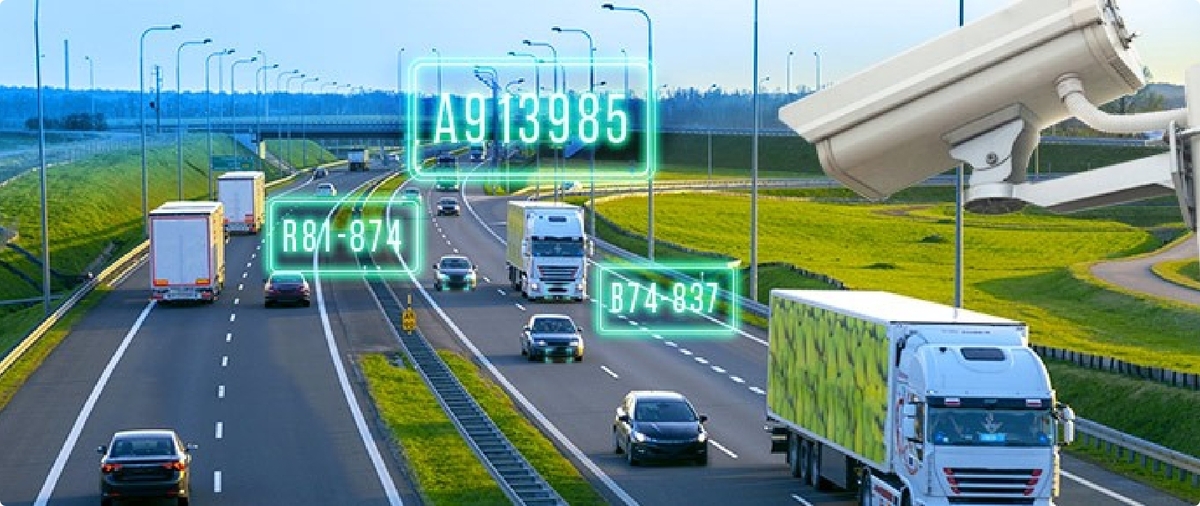
In an interview with Iranian media, Farideh Sadat Jamali, whose Master’s thesis in the field of mechatronic engineering was used to produce the plate detection system pointed to some of the challenges when detecting license plates, saying “Enough light to detect the plates numbers, weather conditions, angle of view of the camera, the number of license plates in the image, the presence of an object similar to the license plate, the presence of an obstacle or distorted license plate, forgery of plate, etc. are among the uncontrollable challenges that the vehicle license plate detection systems face.”
“In this research, in addition to designing and implementing a real-time model for automatic license plate detection, a model for car model detection has also been designed. In this way, the plate number detection error is reduced as much as possible and the cases caused by wrong plate detection are also avoided,” she added.
“Considering the progress that has made in the field of deep learning and neural networks, convolutional neural networks have been used in this research. In the proposed method of this research, car number plate detection and car model detection are both done in two stages. To detect the license plate in the first step, the rectangle of the license plate is recognized in the input image. Then, the license plate is separated from the input image and in the next step, the license plate identifiers are detected. For both stages of this section, YOLO models based on convolutional neural network have been used,” Jamali said.
She added that her method has yielded good results with an average accuracy of 95.5% and an inference time of 43.3 milliseconds on the computer.
“The proposed model for real-time detection of license plates has been implemented on the advanced Jetson Nano board, and its inference speed is estimated at 6 frames per second," the engineer went on to say.
Detecting the car model is done in two steps, according to the researcher.
"First is detecting the front view of the car and next detecting the car model from the cropped image of the previous step,"She said, adding, “For the first stage of this process, the Yolo model has been used, and for the final detection of the car model, the Siamese Convolutional Neural Network with appropriate executive features has been used. This model also yielded acceptable results with an average accuracy of 92.2% and a response time of 85.6 milliseconds on the computer.”
4155/i